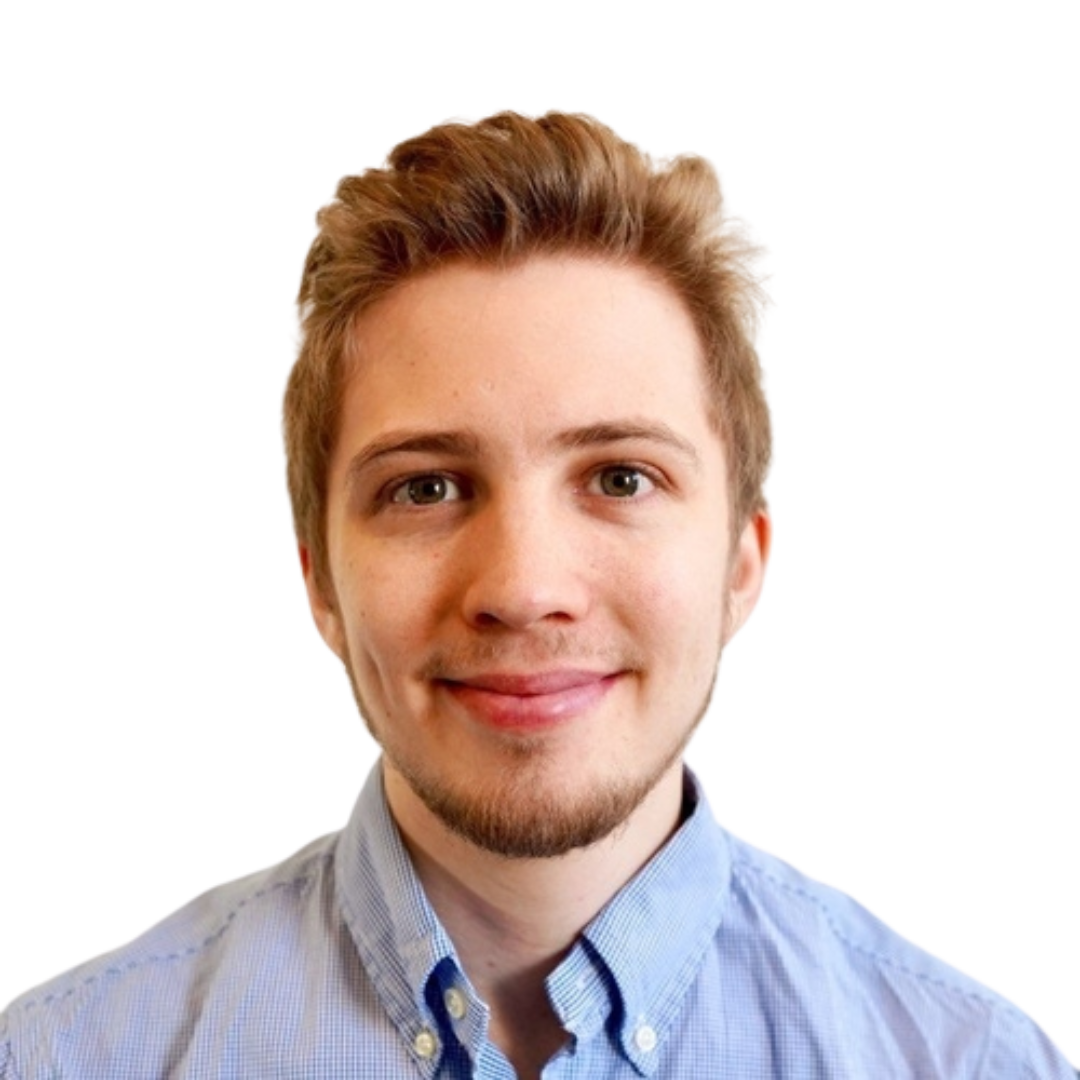
From McKinsey to technical AI safety (with a focus on impact)
We caught up with Constantin Weisser, an ex-McKinsey data scientist who recently started as a technical staff member at Haize Labs doing safety testing for large language models. Constantin shares his insights on making the leap from consulting to a high-impact role in AI safety, and how his experience at McKinsey shaped his career trajectory.
Why leave McKinsey?
AI is growing massively, and with it, both the potential benefits and drawbacks are increasing significantly. At McKinsey, I had a beautiful three years and two months of training. I gained skills in understanding complex problems across six different industries and provided real business value through AI. On the technical side, I learned about developing production systems and working with complicated codebases. I also learned a lot about how organizations function and how to nurture products from infancy to growth.
However, I was always looking for a way to give back to the community and make a positive impact on the world. As I progressed in my career at McKinsey, I reached a fork in the road. I could either continue down this path for a long time, potentially giving up some of my technical background to oversee projects, or I could leave to stay technical and make an impact in other ways. I realized it was time to make the jump.
The field of AI safety has evolved from a niche industry to one where empirical work can drive significant impact. There's a clear need for good evaluations, novel tests, and red teaming - things that society knows we should invest in but haven't received enough support. The biggest pull factor was my desire to make AI systems safer, knowing that my time at McKinsey had prepared me well to drive real impact in this field.
How did your learning and growth evolve at McKinsey?
I was still learning and growing at McKinsey, but I noticed that my learning wasn't directed in the way I wanted. As the problem of AI safety became clearer to me, I realized that the skills I was acquiring - while good to have in general - were not necessarily preparing me in the optimal way to have an impact in this specific field.
Earlier in my time at McKinsey, I was learning crucial skills like how to structure projects from the ground up. These are valuable for having an impact in almost any field. However, as time went on, I found myself acquiring more generic skills.
As I understood the AI safety community better, I realized I could play a significant role and have a positive impact. This realization, combined with my evolving skill set, led me to make the transition.
What steps did you take to make your transition easier?
The transition wasn't easy. As a data science consultant jumping into technical AI safety, I had to prepare thoroughly.
I knew I needed to secure a good job or learning opportunity within AI safety before leaving McKinsey. This meant a lot of self-studying and understanding of the main opportunities in the field. The MATS (Machine Learning for Alignment Theory Scholars) program was a great opportunity for me. It allowed me to take a summer off from McKinsey to try out this new field, see if I liked it, and develop skills that would make the jump into an AI safety job significantly easier.
I also made use of McKinsey's "Take Time" option, where you can get paid 10-20% less but receive more time off. I used some of that time for self-study.
Additionally, I found like-minded people within McKinsey to discuss these topics and get feedback on what I was learning.
When applying for jobs, I created a spreadsheet to analyze the likelihood of getting different positions, the effort required to apply, and how much I'd like each job. This allowed me to be strategic and focus my efforts on the most promising opportunities.
What would you do differently if you could go back?
Looking back, the main thing I would change is starting my transition earlier and embedding myself into the communities I wanted to enter.
For example, in Boston, there are communities like MAIA and AI Safety Student Team (the MIT and Harvard Student Associations for AI Safety, respectively). It would have been helpful to get to know these groups earlier.
However, given the demanding nature of consulting hours, there wasn't much more I could have realistically done. Finding some time off was crucial for me to prepare properly. Nothing really prepared me for the job interviews and positions as well as just jumping right in.
While my transition might seem drastic in some ways, in others it wasn't. I was still coding and working in data science and machine learning. The biggest changes were in the community, the people I was working with, and the different attitudes towards work.
How do the working styles differ between consulting and AI safety research?
The working styles are quite different. In consulting, we learn to be effective in certain ways. For example, starting meetings with an agenda is a basic but crucial practice that many in research might not appreciate as much. There are also practices like having a single assigned owner for each task.
One thing I learned is that while it's helpful to offer these lessons from consulting, it's important not to force them upon others. You can take initiative and demonstrate the positive impact of these practices, but you can't force people to adopt them.
Consulting often focuses on how things look and how they're perceived, while in research roles, the product itself is almost all that matters. This has pros and cons. On one hand, you can skip some of the political discussions about presentation and focus on the facts. On the other hand, sometimes good ideas don't make it to execution because people forget about that last mile of making it accessible and presentable.
Lastly, I'm taking significantly fewer calls now than I did in consulting. In my current role, it's not as important to get buy-in from everybody, which frees me up to do more technical work.
What advice would you give to consultants considering transitioning to AI safety or other high-impact fields?
One of the most helpful things I did was participate in the BlueDot AI Safety Fundamentals course. There's a technical track and a governance track. Importantly, it puts you in a room (even if it's virtual) with other like-minded people to discuss these topics in a relatively low-stakes environment once a week.
My advice would be to get yourself out there and immerse yourself in the communities you're interested in. Being part of the community ensures that you're working on the right things and upskilling in the right ways. It's easy to fall into the trap of upskilling in some vague way that seems cool but isn't actually practical or helpful. Community involvement helps you avoid that pitfall and provide you the kind of feedback you need to increase your impact over time.